All posts by Editor
Bulk measurement and verification
Anyone familiar with the principles of monitoring and targeting (M&T) and measurement and verification (M&V) will recognise the overlap between the two. Both involve establishing the mathematical relationship between energy consumption and one or more independently-variable ‘driving factors’, of which one important example would be the weather expressed numerically as heating or cooling degree days.
One of my clients deals with a huge chain of retail stores with all-electric services. They are the subject of a rolling refit programme, during which the opportunity is taken to improve energy performance. Individually the savings, although a substantial percentage, are too small in absolute terms to warrant full-blown M&V. Nevertheless he wanted some kind of process to confirm that savings were being achieved and to estimate their value.
My associate Dan Curtis and I set up a pilot process dealing in the first instance with a sample of a hundred refitted stores. We used a basic M&T analysis toolkit capable of cusum analysis and regression modelling with two driving factors, plus an overspend league table (all in accordance with Carbon Trust Guide CTG008). Although historical half-hourly data are available we based our primary analysis on weekly intervals.
The process
The scheme will work like this. After picking a particular dataset for investigation, the analyst will identify a run of weeks prior to the refit and use their data establish a degree-day-related formula for expected consumption. This becomes the baseline model (note that in line with best M&V practice we talk about a ‘baseline model’ and not a baseline quantity; we are interested in the constant and coefficients of the pre-refit formula). Here is an example of a store whose electricity consumption was weakly related to heating degree days prior to its refit:
Cusum analysis using this baseline model yields a chart which starts horizontal but then turns downwards when the energy performance improves after the refit:
Thanks to the availability of half-hourly data, the M&T software can display a ‘heatmap’ chart showing half-hourly consumption before, during and after the refit. In this example it is interesting to note that savings did not kick in until two weeks after completion of the refit:
Once enough weeks have passed (as in the case under discussion) the analyst can carry out a fresh regression analysis to establish the new performance characteristic, and this becomes the target for every subsequent week. The diagram below shows the target (green) and baseline (grey) characteristics, at a future date when most of the pre-refit data points are no longer plotted:
A CTG008-compliant M&T scheme retains both the baseline and target models. This has several benefits:
- Annual savings can be projected fairly even if the pre- or post-refit periods are less than a year;
- The baseline model enables savings to be tracked objectively: each week’s ‘avoided energy consumption’ is the difference between actual consumption and what the baseline model yielded as an estimate (given the prevailing degree-day figures); and
- The target model provides a dynamic yardstick for ongoing weekly consumptions. If the energy-saving measures cease to work, actual consumption will exceed what the target model predicts (again given the prevailing degree-day figures). See final section below on routine monitoring.
I am covering advanced M&T methods in a workshop on 11 September in Birmingham
A legitimate approach?
Doing measurement and verification this way is a long way off the requirements in IPMVP. In the circumstances we are talking about – a continuous pipeline of refits managed by dozens of project teams – it would never be feasible to have M&V plans for every intervention,. Among the implications of this is that no account is taken (yet) of static factors. However, the deployment of heat-map visualisations means that certain kinds of change (for example altered opening hours) can be spotted easily, and others will be evident. I would expect that with the sheer volume of projects being monitored, my client will gradually build up a repertoire of common static-factor events and their typical impact. This makes the approach essentially a pragmatic one of judging by results after the event; the antithesis of IPMVP, but much better aligned to real-world operations.
Long-term routine monitoring
The planned methodology, particularly when it comes to dealing with erosion of savings performance, relies on being able to prioritise adverse incidents. Analysts should only be investigating in depth cases where something significant has gone wrong. Fortunately the M&T environment is perfect for this, since ranked exception reporting is one of its key features. Every week, the analyst will run the Overspend League Table report which ranks any discrepancies in descending order of apparent weekly cost:
Any important issues are therefore at the top of page 1, and a significance flag is also provided: a yellow dot indicating variation within normal uncertainty bands, and a red dot indicating unusually high deviation. Remedial effort can then be efficiently targeted, and expected-consumption formulae retuned if necessary.
Monitoring external lighting
The diagram below shows the relationship, over the past year, between weekly electricity consumption and the number of hours of darkness per week for a surface car park. It is among the most consistent cases I have ever seen:
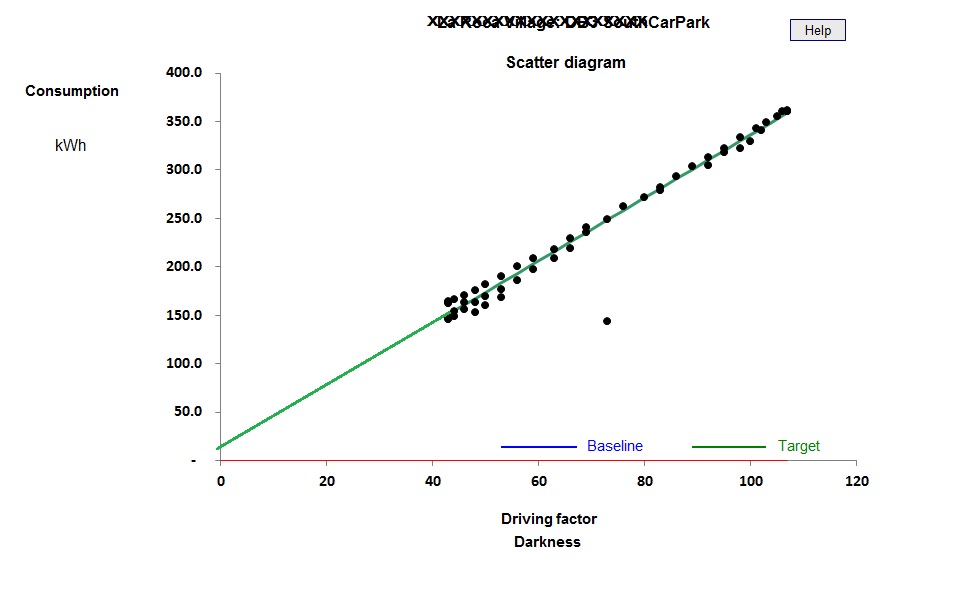
There is a single outlier (caused by meter error).
Although both low daylight availability and cold weather occur in the winter, heating degree days cannot be used as the driving factor for daylight-linked loads. Plotting the same consumption data against heating degree days gives a very poor correlation:
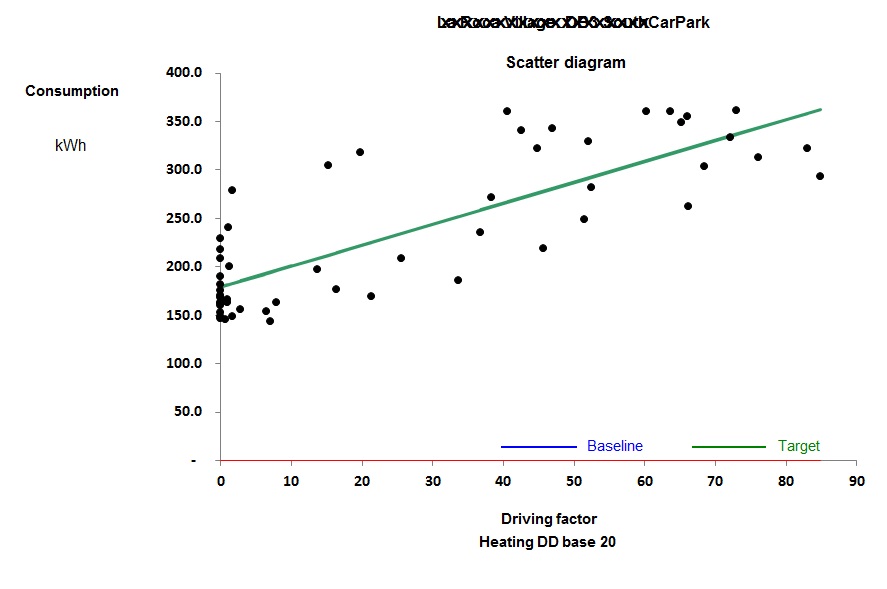
There are two reasons for the poor correlation. One is the erratic nature of the weather (compared with very regular variations in daylight availability) and the other is the phase difference of several weeks between the shortest days and the coldest weather. If we co-plot the data from Figure 2 as a time-series chart we see this illustrated perfectly. In Figure 3 the dots represent actual electricity consumption and the green trace shows what consumption was predicted by the best-fit relationship with heating degree days:
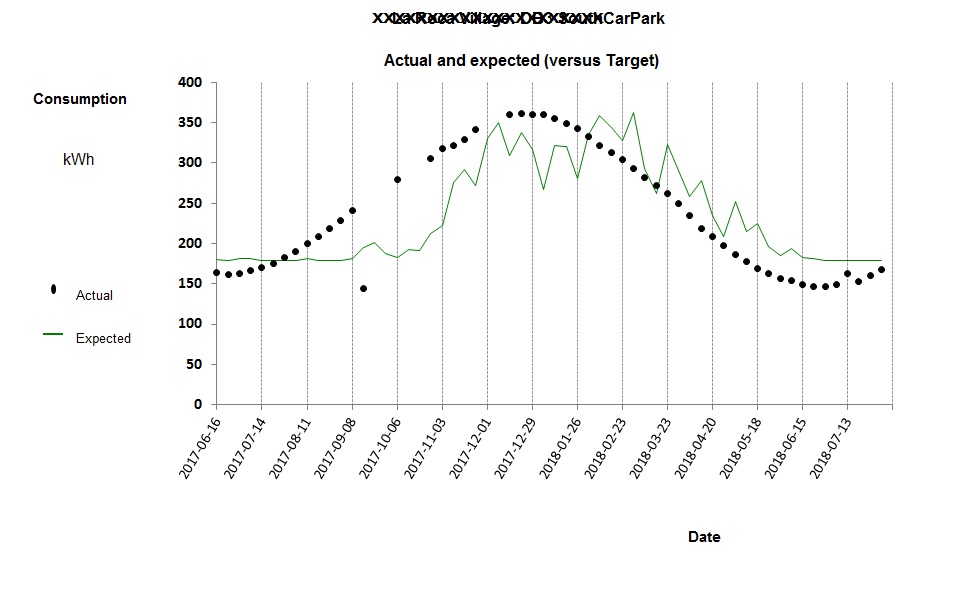
Compare Figure 3 with the daylight-linked model:
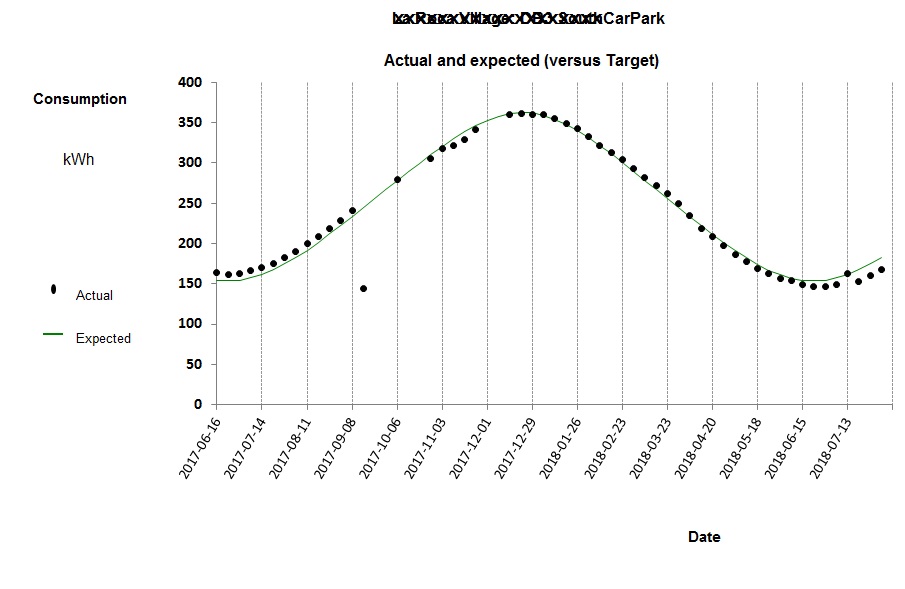
One significant finding (echoed in numerous other cases) is that it is not necessary to measure actual hours of darkness: standard weekly figures work perfectly well. It is evident that occasional overcast and variable cloud cover do not introduce perceptible levels of error. Moreover, figures for UK appear to work acceptably at other latitudes: the case examined here is in northern Spain (41°N) but used my standard darkness-hour table for 52°N.
You can download my standard weekly and monthly hours-of-darkness tables here.
This article is promoting my advanced energy monitoring and targeting workshop in Birmingham on 11 September
ISO 50001: transition to 2018 edition
Project sketch: vetting product offers
My client in this case is an international hotel brand. Individual hotels get approached by people selling questionable energy-saving products and rarely if ever have enough knowledge to defend themselves against bogus and exaggerated offers.
The company has established a core group of engineers and sustainability staff to carry out centralised vetting. My job is to provide technical advice during the initial filtering phase and to join a twice-yearly meeting to interview suppliers who are being taken further.
Project sketch: user requirement specification
Our client, a university, has a long-established metering system based on proprietary hardware with associated software for managing and interrogating the meters and storing their output for use, among other things, in a monitoring and targeting scheme. They have two major stakeholders, one predominantly interested in monitoring and managing power quality and availability, and the other in billing the various user departments. The existing scheme suffers from certain limitations and the client is considering migrating to a new data-collection provider. Continue reading Project sketch: user requirement specification
Project sketch: bulk measurement and verification
Our client in this case is a national retail chain which is continually and progressively improving its estate through the application of generic energy-saving fixes. Savings need to be measured and verified, but individual project values and expected savings are generally too low to merit the cost of rigorous adherence to the International Performance Verification Protocol.
We are conducting a proof-of-concept study Continue reading Project sketch: bulk measurement and verification
Project sketch: dynamic budgeting
Our client, a commercial landlord operating business parks in various major European cities, wants to tighten up budgetary control by accurately profiling the forecast monthly consumptions and expenditures on about 30 electricity accounts.
Using M&T techniques on billing patterns
One of my current projects is to help someone with an international estate to forecast their monthly energy consumption and hence develop a monthly budget profile. Their budgetary control will be that much tighter because it has seasonality built in to it in a realistic fashion.
Predicting kWh consumptions at site level is reasonably straightforward because one can use regression analysis against appropriate heating and cooling degree-day values, and then extrapolate using (say) ten-year average figures for each month. The difficulty comes in translating predicted consumptions into costs. To do this rigorously one would mimic the tariff model for each account but apart from being laborious this method needs inputs relating to peak demand and other variables, and it presumes being able to get information from local managers in a timely manner. To get around these practical difficulties I have been trying a different approach. Using monthly book-keeping figures I analysed, in each case, the variation in spend against the variation in consumption. Gratifyingly, nearly all the accounts I looked at displayed a straight-line relationship, i.e., a certain fixed monthly spend plus a flat rate per kWh. Although these were only approximations, many of them were accurate to half a percent or so. Here is an example in which the highlighted points represent the most recent nine months, which are evidently on a different tariff from before:
I am not claiming this approach would work in all circumstances but it looks like a promising shortcut.
Cusum analysis also had a part to play because it showed if there had been tariff changes, allowing me to limit the analysis to current tariffs only.
The methods discussed in this article are taught as part of my energy monitoring and targeting courses: click here for details
Furthermore, in one or two instances there were clear anomalies in the past bills where spends exceeded what would have been expected. This suggests it would be possible to include bill-checking in a routine monitoring and targeting scheme without the need for thorough scrutiny of contract tariffs.
Review of ISO50001:2018
I ALWAYS THOUGHT that the diagrammatic representation of the “plan, do, check, act” cycle in ISO50001:2011 was a little strangely drawn (left-hand in picture below), although it does vaguely give the sense of a preparatory period followed by a repetitive cycle and occasional review. Turns out, though, that it was wrong all along because in the 2018 version of the Standard, the final draft of which is available to buy in advance of publication in August, it seems to have been “corrected” (right-hand below). For my money the new version is less meaningful than the old one.
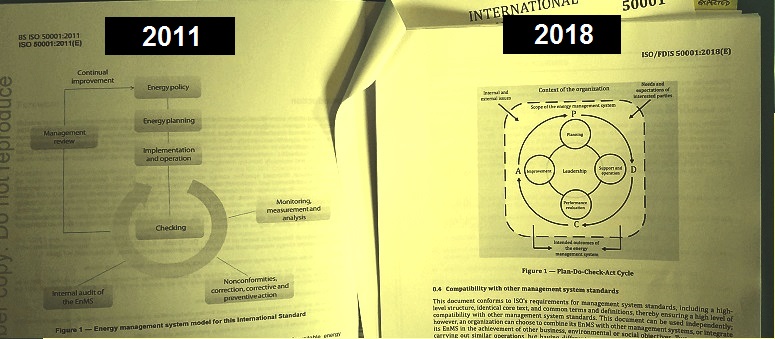
ISO50001 has been revised not because there was much fundamentally wrong with the 2011 version but as a matter of standards policy: it and other management-system standards such as ISO9001 (quality) and ISO14001 (environment) have a lot in common and are all being rewritten to match a new common “High Level Structure” with identical core text and harmonized definitions. ISO50001’s requirements, with one exception, will remain broadly the same as they were in 2011.
It is just a pity that ISO50001:2018 fails in some respects to meet its own stated objective of clarity, and there is evidence of muddled thinking on the part of the authors. The PDCA diagram is a case in point. I see also, for example, that the text refers to relevant variables (i.e., driving factors like degree days etc) affecting energy ‘performance’ whereas what they really affect is energy consumption. To take a trivial example, if you drive twice as many miles one week as another, your fuel consumption will be double but your fuel performance (expressed as miles per gallon) might well be the same. Mileage in this case is the relevant variable but it is the consumption, not the performance, that it affects. This wrong-headed view of ‘performance’ pervades the document and looking in the definitions section of the Standard you can see why: to most of us, energy performance means the effectiveness with which energy is converted into useful output or service; ISO50001:2018 however defines it as ‘measurable result(s) related to energy efficiency, energy use, and energy consumption’. I struggle to find practical meaning in that, and I suspect the drafting committee members themselves got confused by it.
Furthermore, the committee have ignored warnings about ambiguity in the way they use the term Energy Performance Indicator (EnPI). There are always two aspects to an EnPI: (a) the method by which it is calculated—what we might call the EnPI formulation—and (b) its numerical value at a given time. Where the new standard means the latter, it says so, and uses the phrase ‘EnPI value’ in such cases. However, when referring to the EnPI formulation, it unwisely expresses this merely as ‘EnPI’, which is open to misinterpretation by the unwary. For example Section 6.4, Energy Performance Indicators, says that the method for determining and updating the EnPI(s) shall be maintained as documented information. I bet a fair proportion of people will take the phrase ‘determining and updating the EnPI(s)’ to mean calculating their values. It does not. The absence of the word ‘values’ means that you should be determining and updating what EnPIs you use and how they are derived.
Failure to explicitly label EnPI ‘formulations’ as such has also led to an error in the text: section 9.1.1 bullet (a) (2) says that EnPIs need to be monitored and measured. That should obviously have said EnPI values.
The new version adds an explicit requirement to ‘demonstrate continual energy performance improvement’. No such explicit requirement appeared in the 2011 text, but since last year thanks to the rules governing certifying bodies, you cannot even be certified in the first place if you don’t meet this requirement. There was a lot of debate on this during consultation, but this new requirement survived even though it does not appear in the much-vaunted High Level Structure which ISO50001 was rewritten supposedly to conform to. That being the case, it is paramount that users adopt energy performance indicators that accurately reflect progress. Simple ratio-based metrics like kWh/tonne (or in data centres, Power Usage Effectiveness) are not fit for purpose and their users risk losing their certification because EnPIs of that kind often give perverse results and may fail to reflect savings that have really been achieved.
On a positive note, the new version of the Standard retains the requirement to compare actual and expected energy consumption, and to investigate significant deviations in energy performance. These requirements are actually central to effective ongoing energy management. Moreover, a proper understanding of how to calculate expected consumption is the key to the computation of accurate EnPIs, making it a mission-critical concept for anyone wanting to keep their certification.
This article is promoting my training courses on energy monitoring and targeting which include (a) the use of consumption data to detect hidden waste and (b) how to derive meaningful energy performance indicators.
This review is based on the Final Draft of ISO50001:2018 which has been released on sale prior to formal publication in August 2018.